The article discusses the issue of bias in algorithmic decision-making and its implications in creating an equitable future driven by artificial intelligence (AI). It emphasizes the need to address biases in AI systems to ensure fair and unbiased decision-making processes. The article highlights that biases can be unintentionally embedded in algorithms due to the data used for training, leading to discriminatory outcomes.To mitigate bias in AI systems, the article suggests several strategies. One approach is to improve the diversity and representativeness of the data used for training algorithms. This involves considering various factors such as race, gender, and socioeconomic background to ensure a fair representation of different groups. Additionally, the article emphasizes the importance of transparency in algorithmic decision-making. Making the decision-making process more transparent can help identify and address bias more effectively.Furthermore, the article calls for responsible AI governance, which involves collaboration between policymakers, AI developers, and stakeholders to establish guidelines and regulations that ensure fairness and accountability in AI systems. The article concludes that addressing bias in algorithmic decision-making is crucial to pave the way for an equitable AI-driven future.
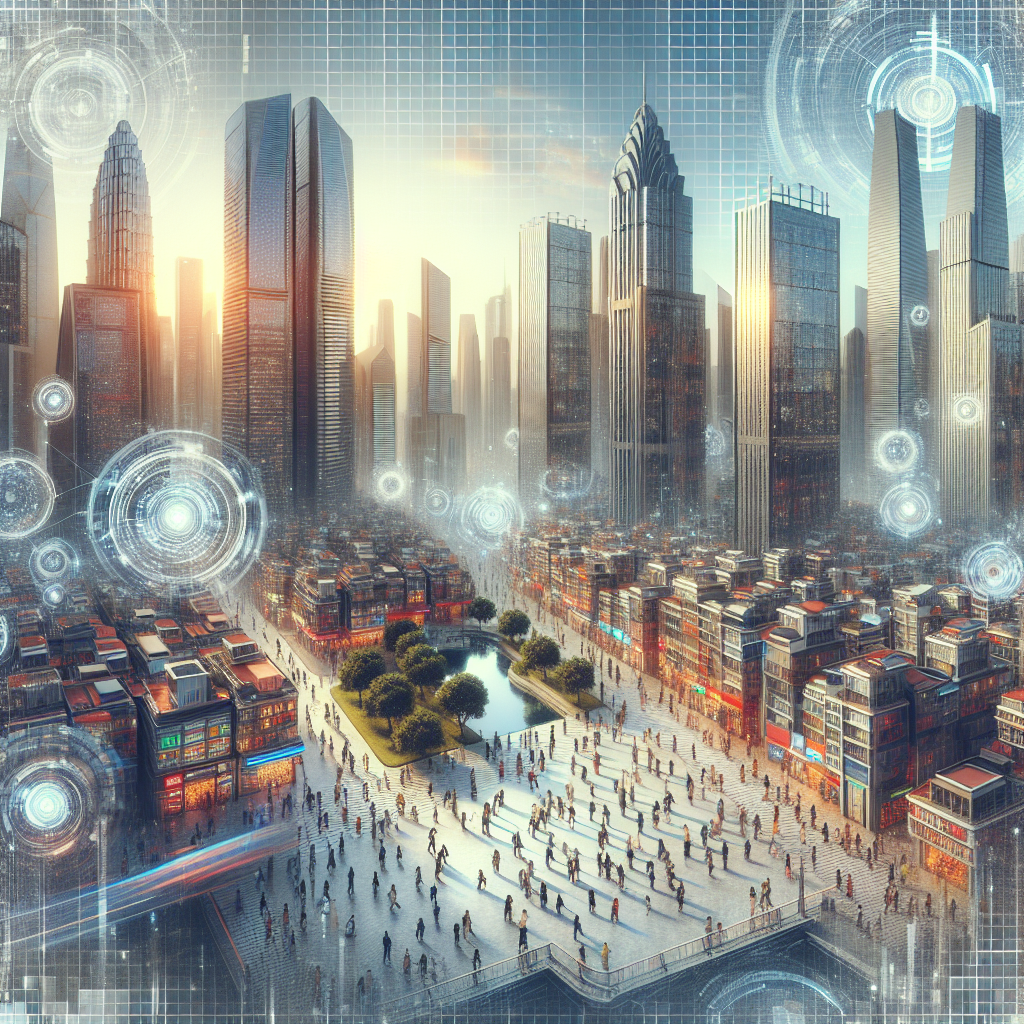
Understanding Bias in Algorithmic Decision-Making
Algorithmic decision-making forms the crux of numerous digital experiences today. It influences a wide range of sectors, from personalized recommendations on Netflix to the tailored advertisements on social media platforms. In essence, algorithmic decision-making is the process of making determinations or predictions based on a set of data using automated, mathematical models.
However, a pressing issue concerning this concept is the potential for bias. Bias in algorithmic decision-making occurs when these automated models, intentionally or unintentionally, favor one group or outcome over another. This could be due to skewed data, biased programming, or other factors that distort the objective nature of these algorithms. To understand how these biases can creep into AI systems, it's important to understand how computers were trained to understand natural language, as explained in this article.
The Importance of Mitigating Bias
The implications of bias in algorithmic decision-making are immense and far-reaching, particularly as AI and machine learning technologies continue to permeate our daily lives. Biased algorithms can reinforce harmful stereotypes and perpetuate inequality. For instance, a recruitment algorithm trained on data from a predominantly male workforce may inadvertently disadvantage female candidates.
Therefore, mitigating bias in algorithmic decision-making is not just an ethical imperative but also crucial for ensuring the effective and equitable functioning of AI systems. It involves identifying and rectifying the sources of bias, whether in the data, the model, or the overall system design. This article provides some tips on creating an effective knowledge base, which can be a useful tool in identifying and rectifying biases.
Paving the way for an Equitable AI-Driven Future
In order to establish an equitable AI-driven future, it is essential to implement strategies that actively counteract algorithmic bias. This could involve diversified data collection, transparent AI models, or even legislation to regulate algorithmic decision-making. By addressing this issue head-on, we can work towards a future where AI not only enhances our digital experiences but also upholds the values of fairness and equality. This article provides a playbook for successfully implementing AI in business operations, including strategies for mitigating algorithmic bias.
A Deeper Dive into Bias in Algorithmic Decision-Making
Algorithmic bias refers to systematic and repeatable errors that create unfair outcomes, such as privileging one arbitrary group of users over others. It is a direct reflection of the prejudices inherently present in the society that creates the algorithms. Bias in machine learning can be traced back to either the data used to train the models or the decision-making process of the algorithms themselves.
The phenomenon of algorithmic bias is not a new one. It stems from the early days of the Internet, when algorithms were first used to sort through vast amounts of data. At the roots, algorithms were simply mathematical models that followed a set of pre-defined instructions to solve a specific problem. But as these algorithms evolved and began feeding on data to make decisions, they unknowingly began to absorb the biases contained in the data they were consuming.
Today, in a world increasingly driven by AI, we see the impact of algorithmic bias more than ever before. From hiring practices to credit scoring, predictive policing to personalized advertising, bias in algorithmic decision-making can have far-reaching consequences in people's lives. For instance, an algorithmic hiring tool might inadvertently favor male candidates over female ones if it was trained on historical hiring data from an industry dominated by men.
Understanding the underlying systems that power these biased algorithms becomes crucial to mitigating their effects. Algorithms, at their core, are complex mathematical models that use statistical analysis to make predictions or decisions based on input data. When the input data is biased, it leads to biased outputs. The biases can be conscious or unconscious, explicit or implicit, and stem from numerous sources such as the environment, culture, and personal experiences. This article discusses the lifecycle of AI models, which includes the process of data collection that can introduce bias.
Looking at Variations and Expectations
Algorithmic bias can manifest in several ways, some more subtle than others. There's the issue of representation bias where data is not representative of all relevant social groups, leading to skewed results. Prejudice bias occurs when prejudiced human beliefs seep into the algorithms. Measurement bias happens when the data collected is influenced by irrelevant factors.
It's important to understand that mitigating bias in algorithmic decision-making is not about blaming the algorithms or the data, but about acknowledging the inherent human biases that shape them. Only then can we pave the way towards an equitable AI-driven future, and it starts with everyone recognizing and actively working to address these biases.
Having an understanding of how these biases come to be, how they currently affect the world, and the various ways they manifest, is the first step in mitigating bias in algorithmic decision-making. In the next section, we'll discuss some promising methods currently being explored to tackle this issue head-on.
Why People Use Algorithmic Decision-Making
The Benefits
The benefits of utilizing algorithmic decision-making are immense. For one, it increases efficiency by quickening decision-making processes. It's a tool that can analyze massive amounts of data far quicker than any human could. The speed and accuracy of AI decisions can lead to improved productivity and profitability.
Algorithmic decision-making also promotes consistency. When decisions are based on data and logic rather than emotions or subjective opinions, there's a uniformity that's difficult to achieve otherwise.
The Goals
The primary goal of using algorithms in decision-making is to enhance decision quality. By utilizing AI, organizations aim to make informed decisions based on data-driven insights. Moreover, algorithmic decision-making is often employed with the objective of reducing human error. This article explores the impacts and advantages of AI in business, including the role of algorithmic decision-making.
Ways to Implement Algorithmic Decision-Making
Implementing algorithmic decision-making involves several steps. These include identifying decision-making processes that could benefit from AI, acquiring quality data for the algorithm, developing or choosing the right algorithm, and regularly testing and updating the algorithm to ensure optimal performance.
Algorithmic Decision-Making: Limitations and Cons
Despite the numerous benefits, there are also limitations to the use of algorithms in decision-making.
What to Watch Out For
The most significant concern is bias. If the data fed into the algorithm is biased, it will lead to biased decisions, perpetuating systemic inequalities. Moreover, algorithms are not infallible. They can make mistakes, and when they do, the consequences can be severe.
What's Holding It Back
The main challenge hindering the widespread adoption of algorithmic decision-making is the lack of transparency. Algorithms are often black boxes; their decision-making processes aren't easily understandable by humans. This makes it difficult to diagnose and rectify any issues that arise. This article provides insights into conversational AI, a field that heavily relies on algorithmic decision-making and faces similar challenges of transparency.
Overcoming Limitations
While these problems are daunting, it is possible to overcome them. By implementing measures to ensure data quality and algorithmic transparency, and by putting in place robust checks and balances, we can harness the power of algorithmic decision-making while mitigating its risks.
The Future of Algorithmic Decision-Making
What's Over The Horizon
The future of algorithmic decision-making is promising. Advances in machine learning and artificial intelligence promise to make algorithms even more accurate and efficient. There's also growing interest in explainable AI, which aims to make algorithms transparent and understandable.
What to Expect
In the near future, expect to see more organizations adopting algorithmic decision-making. As the technology improves and transparency concerns are addressed, algorithmic decision-making will become an integral part of business strategy.
Preparing for the Future
To prepare for the future, organizations need to start familiarizing themselves with AI and machine learning. Training staff and investing in technology will be key to staying ahead in the AI-driven future. This article discusses how prescriptive analytics, a form of algorithmic decision-making, can enhance financial service delivery, providing a glimpse into the potential future applications of this technology.
Conclusion
Algorithmic decision-making is a powerful tool that can enhance decision quality and efficiency. However, it's not without its challenges. Issues like bias and lack of transparency need to be addressed to ensure that the benefits of algorithmic decision-making can be fully realized. The future is promising, with advances in AI and machine learning paving the way for more accurate and transparent algorithms. By preparing for this future and addressing current challenges, we can pave the way for an equitable AI-driven future.