In the article "Scaling AI: Strategies for Success," the author discusses the challenges and strategies for successfully implementing and scaling artificial intelligence (AI) within an organization. The author begins by highlighting the growing importance of AI in various industries and the potential benefits it can bring, such as increased efficiency and improved decision-making. However, the author also acknowledges that scaling AI is not an easy task, as it requires a well-defined strategy and careful consideration of various factors.The author outlines three key strategies for scaling AI: starting small and focusing on quick wins, investing in data and infrastructure, and building a culture of continuous learning and improvement. By starting small, companies can minimize risks and gain valuable insights for further scaling. Investing in data and infrastructure is crucial, as AI algorithms heavily rely on high-quality data and robust infrastructure to deliver accurate results. Additionally, building a culture of continuous learning and improvement ensures that the organization can adapt to the rapidly evolving AI landscape.Overall, the article emphasizes the importance of a systematic approach to scaling AI, and provides practical strategies that can help organizations navigate the challenges and maximize the benefits of implementing AI technologies.
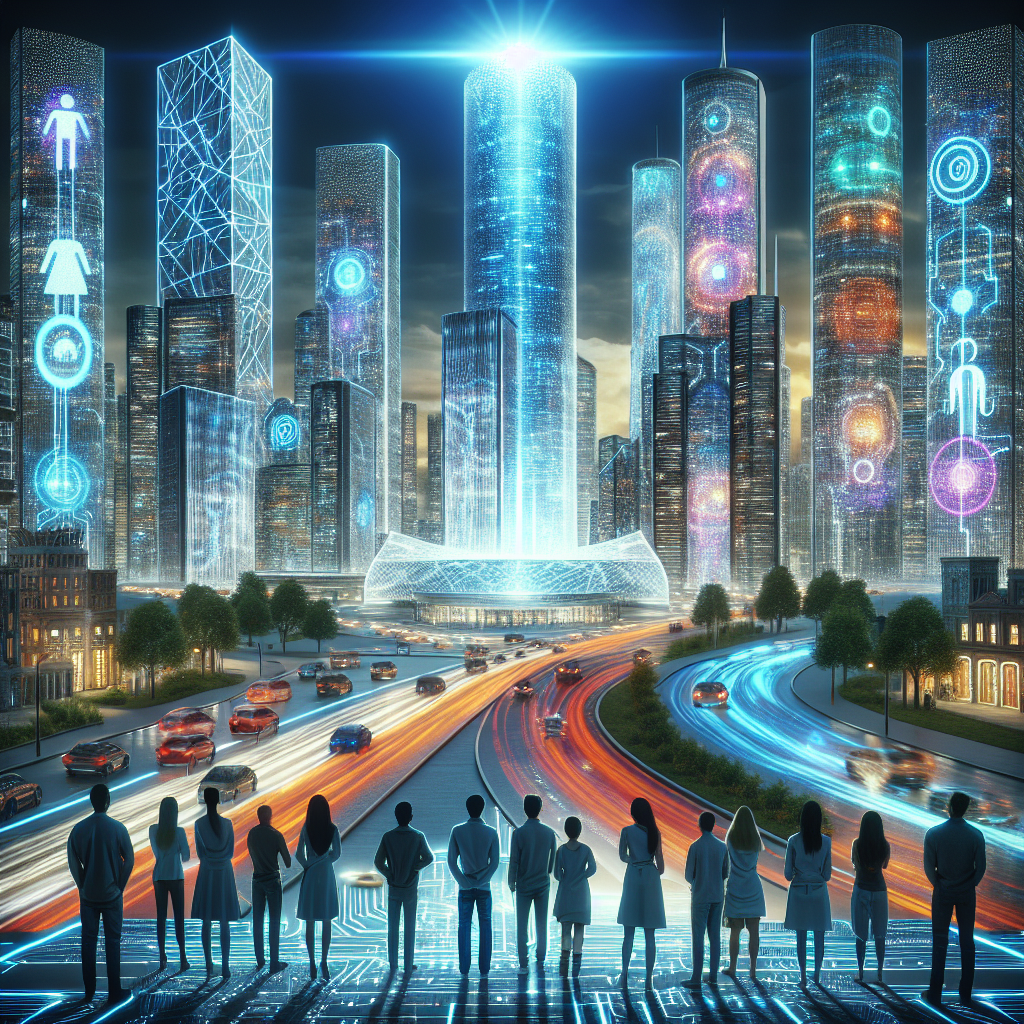
Introduction to Scaling AI: Laying the Foundation
Welcome, AI enthusiasts! Today, we delve into one of the most pivotal aspects of the artificial intelligence realm — scaling AI. This fast-paced technology has seen an exponential rise in its applications, penetrating every industry, from healthcare to entertainment. But to realize its full potential, knowing how to scale AI effectively is of paramount importance.
So, what exactly does 'scaling AI' mean? At its core, scaling AI involves expanding the reach and impact of AI solutions across an organization or infrastructure. It's all about taking a successful AI model or prototype and applying it on a broader scale. This could mean enhancing the model's capacity to handle more data or extending its applications to various business units.
Scaling AI doesn't just involve technical augmentation. It also revolves around aligning AI initiatives with business strategies and fostering an AI-ready culture within an organization. This is where understanding the lifecycle of AI models can be beneficial.
In the subsequent sections, we'll explore various strategies for successfully scaling AI, addressing common challenges, and showcasing real-world examples of successful AI scale-up. Let's embark on this exciting journey together!
A Deeper Dive into the Subject
Artificial Intelligence (AI) is an impressive technological development that's revolutionizing various sectors in our society. However, to maximize its potential, its scalability is imperative. But, what does it mean to scale AI? It refers to the process of expanding the capacity of artificial intelligence systems to handle larger and more complex tasks. This expansion can be in terms of the size of the data being worked with, the complexity of the models or algorithms used, or the computational power of the hardware resources.
How It Works in Depth
Scaling AI involves several technical approaches. One such method is model scaling, which involves growing an existing model's parameters to enhance its performance, such as increasing the depth (more layers), width (more neurons), or resolution (more input). Another approach is data scaling, where more training data are used to improve an AI model's accuracy. Additionally, computational scaling involves increasing the processing power, like utilizing more powerful machines or more machines. Understanding how computers were trained to understand natural language can provide insights into these AI scaling methods.
Tracing the Origins
The concept of scaling AI is not a recent phenomenon. It can be traced back to the early days of AI research, where the need to increase the capacity of AI systems to handle larger tasks was recognized. With advances in computing power, data availability, and algorithmic innovations, the concept of scaling AI has grown in relevance and applicability.
Its Current Role
Today, scaling AI plays a pivotal role in making AI more useful and effective. Companies and organizations can leverage scalable AI to handle more complex tasks and larger data sets, improving their operational efficiency and decision-making capabilities. For instance, in the healthcare sector, scalable AI systems can process massive amounts of patient data to assist in diagnostics and treatment plans. In finance, AI systems can analyze large volumes of market data to provide actionable insights. Here, prescriptive analytics can enhance financial service delivery.
What You Should Know
Understanding the concept of scaling AI and its importance is crucial, especially in today's data-driven world. Scaling AI allows for more advanced and complex problem-solving capabilities. However, it's also important to consider the challenges associated with scaling AI, such as the need for more computational resources and the potential for increased model complexity.
Underlying Systems Powering Scaling AI
The strength of scaling AI lies in its underlying systems. These include powerful computing infrastructure, advanced AI algorithms, and massive datasets. These systems work together to increase the capacity of AI, allowing it to handle larger and more complex tasks efficiently.
Variations and Expectations
While the goal of scaling AI is consistent, the methods and technologies used can vary widely. Depending on the needs and resources of a project, different scaling strategies like model scaling, data scaling, or computational scaling might be used. Regardless of the approach taken, the ultimate goal is to make AI systems more powerful and efficient, capable of handling larger and more complex tasks.
The Advantages and Goals of Scaling AI
The use of Artificial Intelligence (AI) in business and technology has grown exponentially over the past decade. The benefits range from improved efficiency and productivity to the development of new innovative products and services.
Implementing AI at a larger scale offers potential for even greater rewards. Efficiency remains a key driver. With AI, businesses can automate routine tasks, freeing up human resources for more complex and creative tasks. Precision is another significant advantage. AI algorithms can process vast amounts of data with a level of accuracy that would be nearly impossible for humans.
The goal of scaling AI is to integrate this technology into every aspect of business operations, from customer service to product development. To implement this, businesses need a clear strategy. A mix of in-house development and outsourcing, commitment from top leadership, and an organization-wide understanding of AI and its potential are crucial for success. Here, understanding the impacts and advantages of AI in business can be beneficial.
The Challenges of Scaling AI
Despite the potential benefits, scaling AI comes with its own set of challenges. One of the major issues is the lack of skilled professionals. AI requires a specific set of skills and expertise that not all workers possess.
Data privacy is another concern. As AI systems process more data, the risk of privacy breaches increases. Additionally, AI algorithms can sometimes produce biased or discriminatory results, which can lead to legal and ethical issues.
However, these challenges can be overcome. Businesses can invest in training and development to equip their workers with AI skills. They can also implement robust data privacy measures and conduct regular audits to ensure their AI systems are unbiased and fair. Creating an effective knowledge base can be a good starting point for training and development.
The Future of Scaling AI
The future of scaling AI looks promising, with advancements in technology opening up new possibilities. Businesses can expect AI to become even more integrated into their operations, with AI algorithms making more complex decisions and performing more sophisticated tasks. Mastering the art of conversational AI can be a step towards this future.
To prepare for this future, businesses should invest in their technological infrastructure and ensure their workers have the necessary skills. They should also keep a close eye on the latest AI trends and developments.
Conclusion
Scaling AI has the potential to bring about significant benefits, but it also comes with its own set of challenges. However, with careful planning and the right strategies, businesses can successfully implement AI at a larger scale and reap the rewards. As AI technology continues to evolve, businesses need to stay ahead of the curve and prepare for a future where AI is even more integral to their operations.