- RAG (Retrieval-Augmented Generation) is a technique in Generative AI that combines retrieval and generation models to improve content generation capabilities.
- RAG has applications in various industries, including natural language processing, content creation, and image/video generation.
- Benefits of RAG include enhanced contextual understanding and increased efficiency in generating high-quality outputs.
- However, there are challenges and limitations to consider, such as potential biases and ethical concerns in RAG-generated content, data limitations, and computational complexity.
- Future trends and developments in RAG include advancements in research and the integration of RAG with other AI techniques.
- RAG has the potential to make a significant impact on AI technology and various industries in the future.
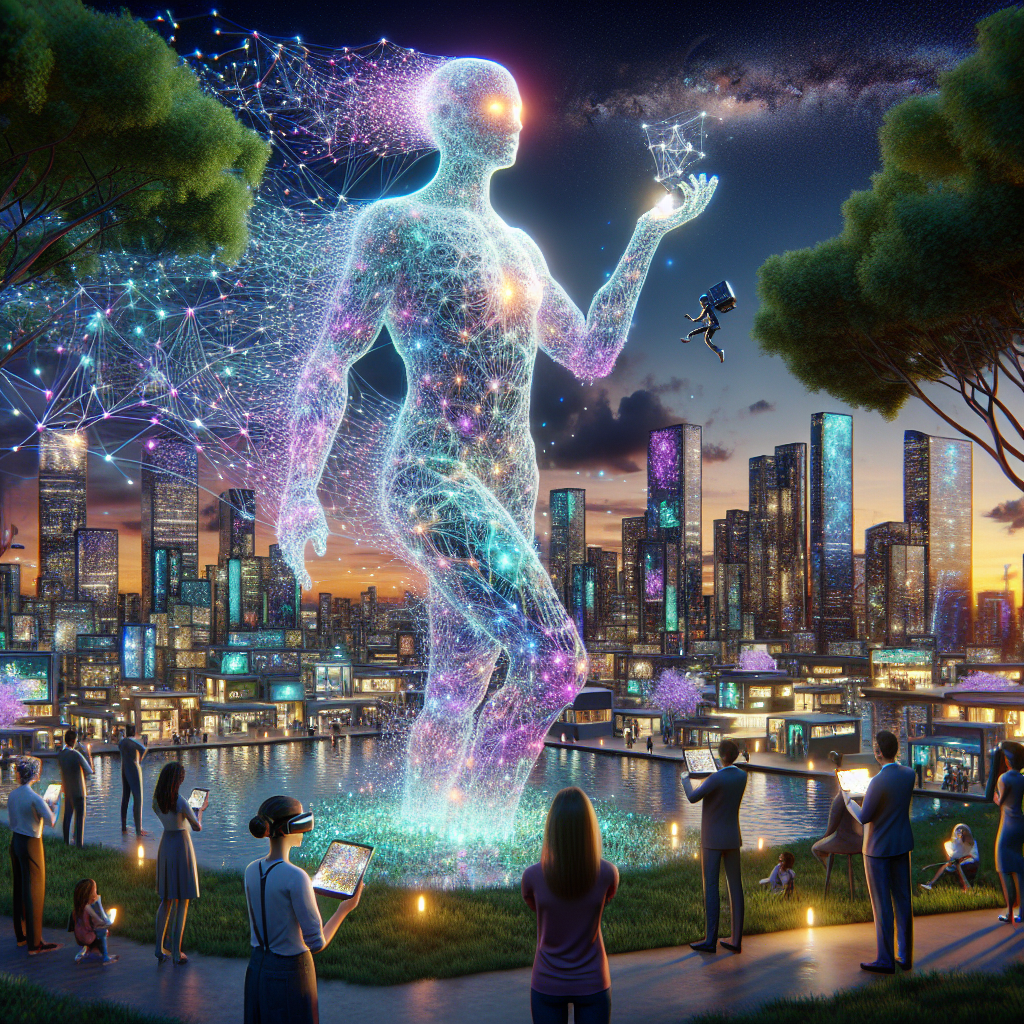
Introduction
Artificial Intelligence (AI) and automation have long been transforming various industries, revamping the way operations are carried out, and changing the face of innovation. Generative AI has been a significant player in this revolution, and one of its most exciting trends is the Rise of Retrieval-Augmented Generation (RAG) that combines the power of retrieval and generation models in a unique and powerful manner. This blog will take you through the groundbreaking world of RAG and how it promises to shape the future of Generative AI.
Understanding RAG in Generative AI
What is RAG?
Retrieval-Augmented Generation (RAG) is a novel approach in the field of Generative AI, amalgamating the strengths of retrieval and generative models. Through a seamless blend of these models, RAG leverages previously learned data and dynamically incorporates it into a generative process. This dual functioning is what sets RAG apart and is sparking a drastic paradigm shift in the Generative AI domain.
Benefits of RAG in Generative AI
The rise of RAG is attributed to the numerous benefits it brings to the table. From high-quality output generation to enhanced contextual understanding, the synergy of retrieval and generative models in RAG offers various advantages. Additionally, RAG boasts of improved content generation capabilities, which leads to their high efficiency in producing superior quality results.
Applications of RAG in Various Industries
With its robust capabilities, RAG is revolutionizing a wide range of industries, bringing unprecedented improvements and innovations.
RAG in Natural Language Processing (NLP)
In the field of Natural Language Processing (NLP), RAG is making significant strides. Its feature to adapt and contextualize uniquely makes it potent for creating sophisticated models for chatbots, language translation, and other language-based AI tasks. Read more about the transformational role of Generative AI in support organization for deeper insights.
RAG in Content Creation
RAG's application extends to content creation, where it's used to generate creative content dynamically. By harnessing the capabilities of RAG, the storytelling and writing processes can be significantly enhanced, leading to a more immersive and engaging user experience. Discover more about how Generative AI can enhance customer satisfaction scores.
To be continued...
In the upcoming sections, we will delve into the applications of RAG in image and video generation, its associated challenges and limitations, and future trends and developments in this field. Stay with us as we unwrap the fascinating realm of RAG in Generative AI.
Challenges and Limitations of RAG in Generative AI
Effective use of AI is never without its fair share of challenges and constraints. The same applies to the use of RAG models in generative AI. In this section, we delve into some of the most prominent challenges, including ethical considerations, data limitations, and computational complexity.
Ethical Considerations
Just like any AI application, RAG is not immune to potential risk of biases creeping into the content it generates. Understanding and mitigating these biases is of paramount importance for ensuring ethically responsible usage of this technology.
Another ethical concern revolves around the transparency of RAG-generated content. As AI becomes more sophisticated, it is imperative that users are made aware of the content generated by AI versus that created by humans. This blog post delves deeper into ethical considerations in AI.
Data Limitations
The quality and quantity of data used to train RAG models is another significant challenge. Availability of high-quality, diverse data ensures a more inclusive and accurate RAG model. However, lack of it could lead to a model that isn't as effective as desired. Here you can learn more about the importance of high-quality data in AI.
Computational Complexity
RAG models, similar to other deep learning models, require substantial computational resources for training and deployment. This includes both processing power and memory requirements, especially for larger models. This computational overhead might present a challenge for organizations with resource constraints.
Future Trends and Developments in RAG
As the field of AI continues to evolve at a rapid pace, it is exhilarating to speculate about what the future might hold for RAG in generative AI. In this section, we discuss potential advancements in RAG research and how RAG is likely to synergize with other AI techniques.
Advancements in RAG Research
Ongoing research and development are pushing the capabilities of RAG, and we might see more significant breakthroughs in the near future. This includes developments that lead to models that are more efficient, capable, and adaptable to various tasks. This blog discusses some of the latest advancements in the field of RAG.
Integration with Other AI Techniques
RAG can be combined with other AI techniques such as reinforcement learning and unsupervised learning for improved performance. Such synergies could lead to fundamentally transformative developments in generative AI. Check out this blog post to learn more about how AI techniques can work hand in hand to create powerful applications.
Conclusion
From improving NLP tasks and revolutionizing content creation to enhancing image and video generation capabilities, RAG has been a significant factor in the rise of generative AI. Despite the challenges, the exciting developments and the potential impact on various industries make RAG an area in AI technology to watch out for!
Now is a great time to dive into the world of RAG in generative AI, and we hope this post provided you with a comprehensive overview. To stay updated with the latest trends in AI, do visit our blog regularly.